Insights
Why Applying Quality Improvement Techniques to Non-clinical Data Makes Sense
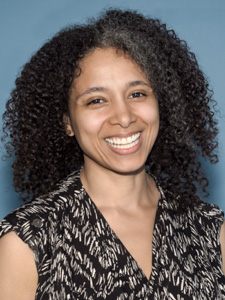
The growing trend of increasing capacity and timeliness of collecting surveillance data (such as birth and death records used by epidemiologists) is opening up opportunities for these rich data sources to be used for quality improvement (QI) efforts. In the Collaborative Improvement and Innovation Network to Reduce Infant Mortality (Infant Mortality CoIIN), states are working towards using preliminary vital records data from birth and death records to better understand infant mortality, as well as the risk factors that contribute to a U.S. infant mortality rate that is almost three times higher than other industrialized countries.
Using surveillance data in QI activities—which calls for small, rapid tests of change—brings a new lens to how this surveillance data can be used. It establishes a demand for increased, real-time monitoring and learning, which can allow for improvements in both policies and programs targeted at improving public health. Encouraging the use of real time data can help state programmatic and policy leaders see the impact of innovative programs, and when necessary make course corrections to make the best use of evidence-based practices. Using a QI lens in public health efforts provides tremendous opportunity for new learning from ongoing efforts to reduce infant mortality and other public health challenges. It provides a new way of looking at data that is already being routinely collected.
To use these tools, it is first important to appreciate some of the differences between how data is analyzed in these two disciplines. Here are four key differences in how QI practitioners and epidemiologist look at data.
- More data over more time periods. QI data is generally examined over time in a run chart. The more points we have, the greater the ability to detect trends, shifts or astronomical data points in our system. While monthly data is often difficult to achieve, we are seeing greater and greater ability to develop quarterly reporting on datasets including vital records, which allows for an increased ability to view data and see variation across time.
- Increasing timeliness of data to support learning. In order to respond to data and learn from interventions and programmatic efforts, programs and policy developers need to be able to see data that is as close to real time as possible. This means increasing data timeliness and in some cases the use of provisional data to begin to learn from the data that is available, rather than waiting for final data, which may take a year or longer to become available.
- Determining an acceptable level of bias. Epidemiological studies are focused on removing as much bias as possible. In QI, the focus is not on removing bias, but ensuring that it remains consistent across time. QI statistics and techniques allows you to account for potential bias and accept its presence without it impacting on the learning from your data. However, completeness of data may still be an issue to consider when determining an acceptable level of bias.
- Accounting for variability is key to both methodologies. In QI, the large number of data points allows us to account for variability in our charts by creating control lines to see if changes in our data are due to a common cause or a special cause. In both QI and epidemiology, the use of statistical methods allows us to help account for variation in our data, and make appropriate interpretations based on our understanding of what our data is showing. Although the methods may differ, the end result is the same as we are able to detect points that fall outside the ‘expected’ results.
QI and epidemiology often look at very similar problems and more and more often are able to make use of the same data. However, these two branches of study utilize different tools to help us understand what is contributing to our outcomes. But it is important to understand that both methodologies are attempting to help us understand cause and effect relationships, and learn from our programmatic efforts how to better implement and understand the impact of interventions to improve health. When we combine these skill sets, we add an important layer of understanding that can only improve our ability to deliver effective and evidence-based programs and policies to improve the health of children.
Sabrina Selk is an Associate Director of Applied Research and Evaluation at NICHQ.